Lin Lab
The Lin Lab, led by Dr. Xihong Lin at the Harvard T.H. Chan School of Public Health, advances genomics and human disease research using innovative statistical and machine learning methods. Our team analyzes large-scale genetic, genomic, and health data to study complex diseases, focusing on areas like whole genome sequencing, functional variant annotation, polygenic risk prediction, and gene-environment interactions. We develop scalable tools, including FAVOR and STAAR, and prioritize improving prediction accuracy for underrepresented populations.
655 Huntington Ave, Boston, MA 02115
Lab Members
Principal Investigator
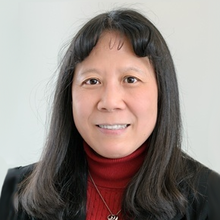
Dr. Lin’s research spans statistical methods, machine learning, and epidemiology, including predictive modeling, causal inference, and COVID-19 studies. A member of the National Academies of Sciences and Medicine, she has received numerous awards, including the COPSS Presidents’ Award and the Marvin Zelen Leadership Award, and has served in prominent roles within national and international statistical organizations.
Research Staff
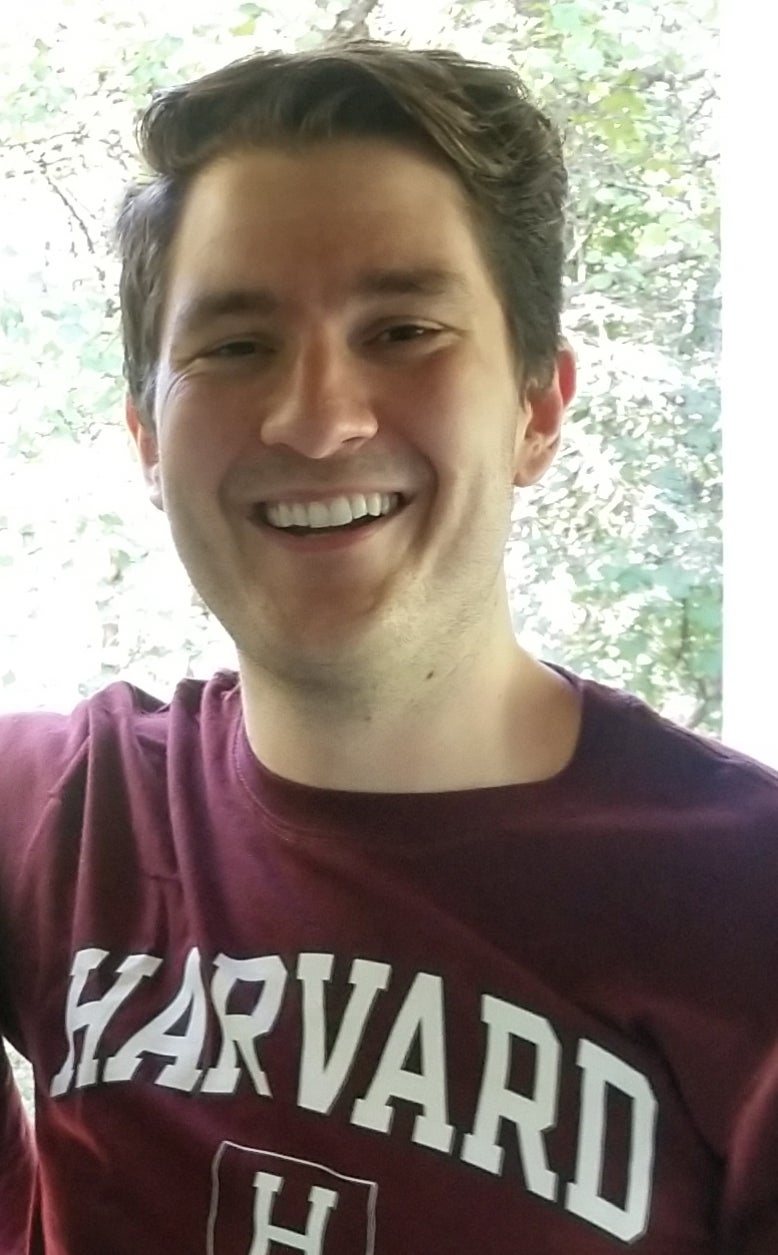
Dr. Van Buren’s primary research focus is the development of new statistical methods for integrative analyses of whole genome sequencing datasets, single-cell sequencing datasets, and multi-omics datasets. He also actively participates in the NHGRI’s IGVF consortium, in which he is working to build a model to predict functional genetic variants from perturbation experiments such as CRISPR and MPRA using functional annotations and variant characterization datasets.
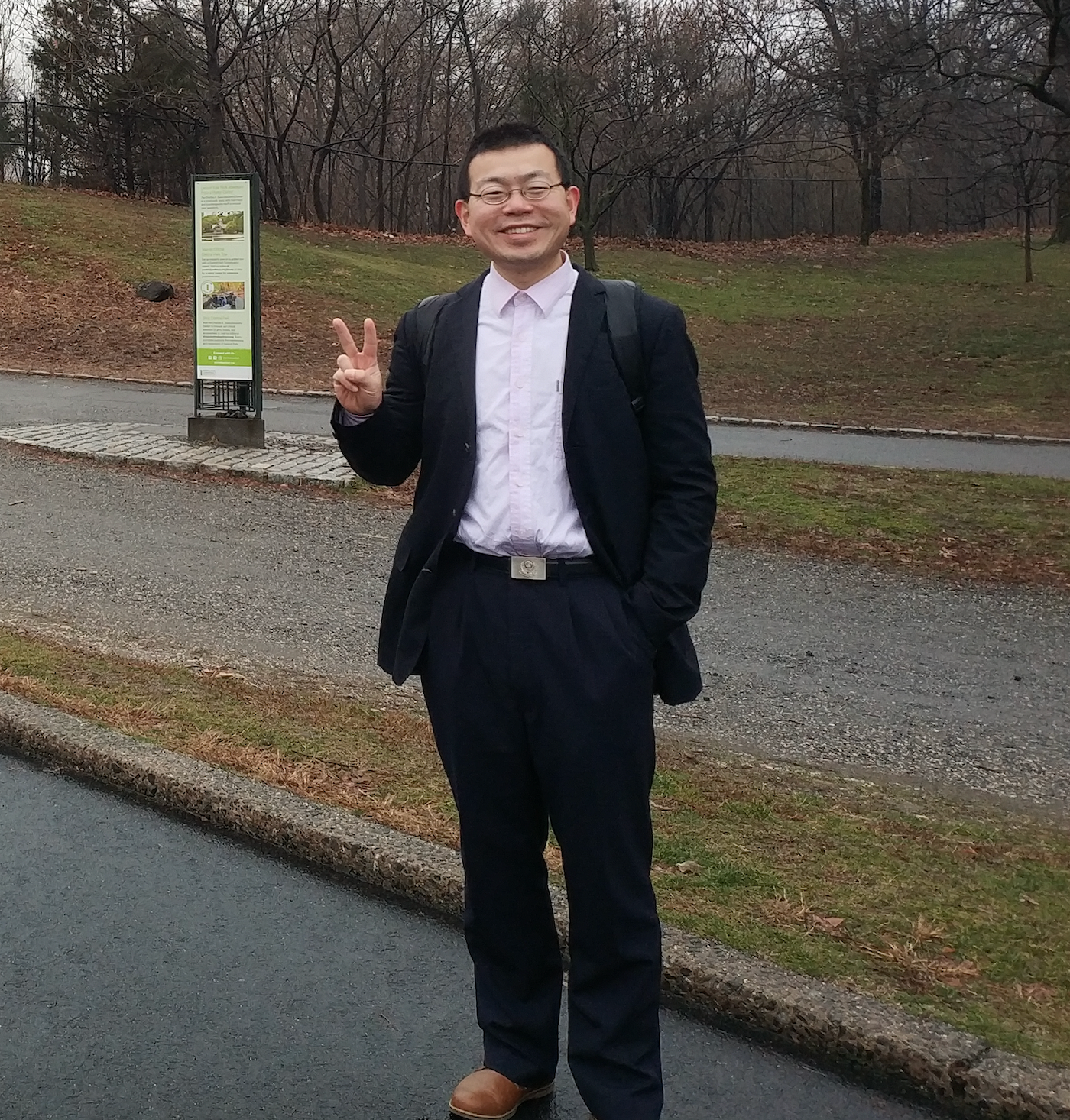
Dr. Zhou’s primary focus is on the functional annotation of genetic variants, building and maintaining annotation databases, and ensuring the quality of large-scale whole-genome sequencing studies. Additionally, he is intrigued by the potential of generative AI to advance genetics research.
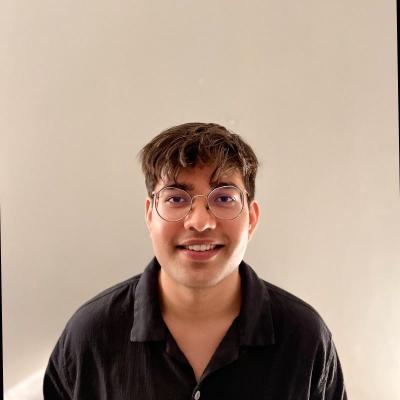
As a software engineer in the Lin Lab, Vineet built genomic applications like FAVOR, managing large-scale datasets and developing web-based visualizations that empower researchers to uncover insights in human genetics.
Postdoctoral Research Fellows
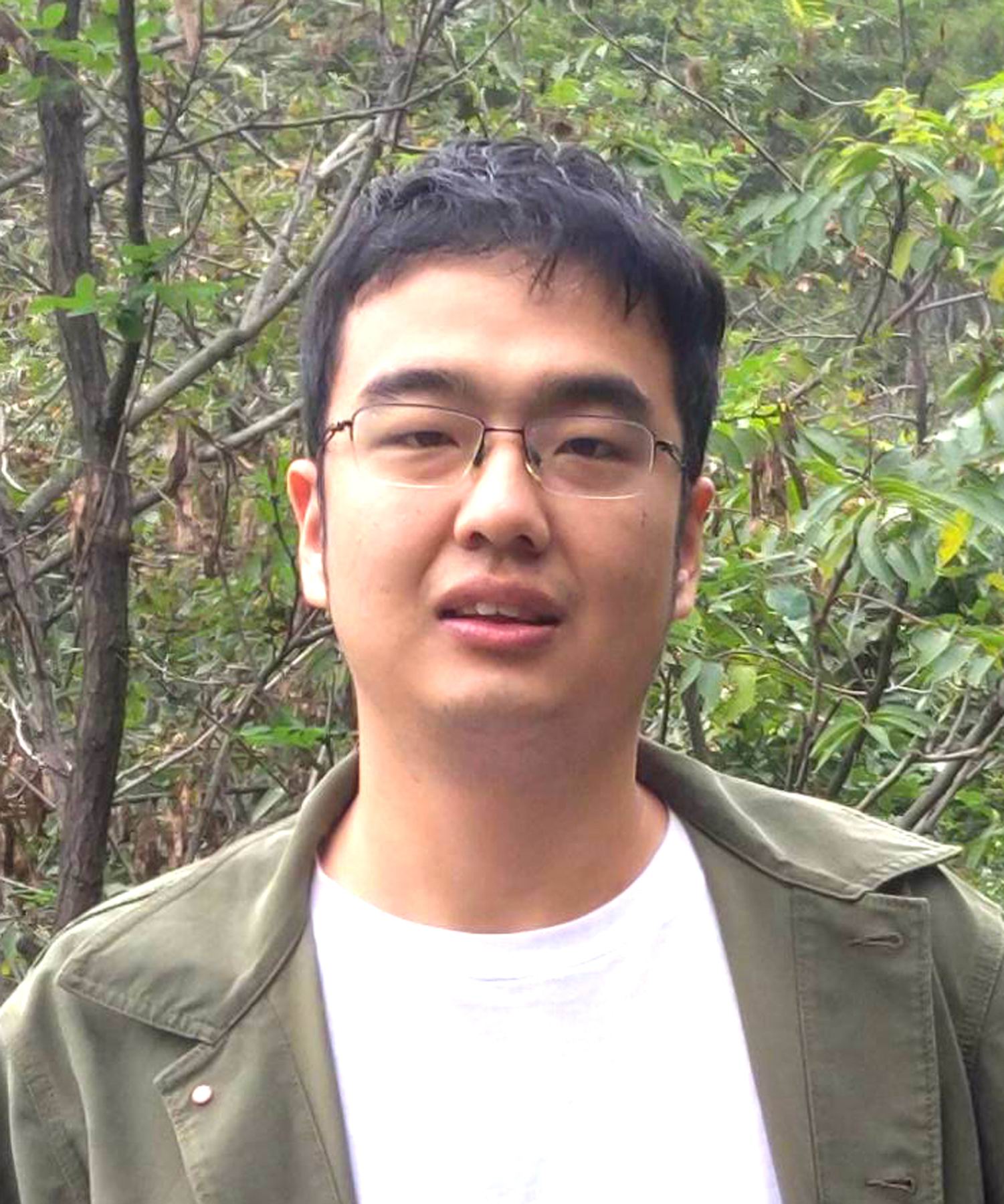
Dr. Wang’s research primarily focuses on developing methodologies for causal inference and data integration. His areas of specialization include Mendelian randomization, large-scale mediation analysis, transfer learning, and data integration for large-scale association studies.
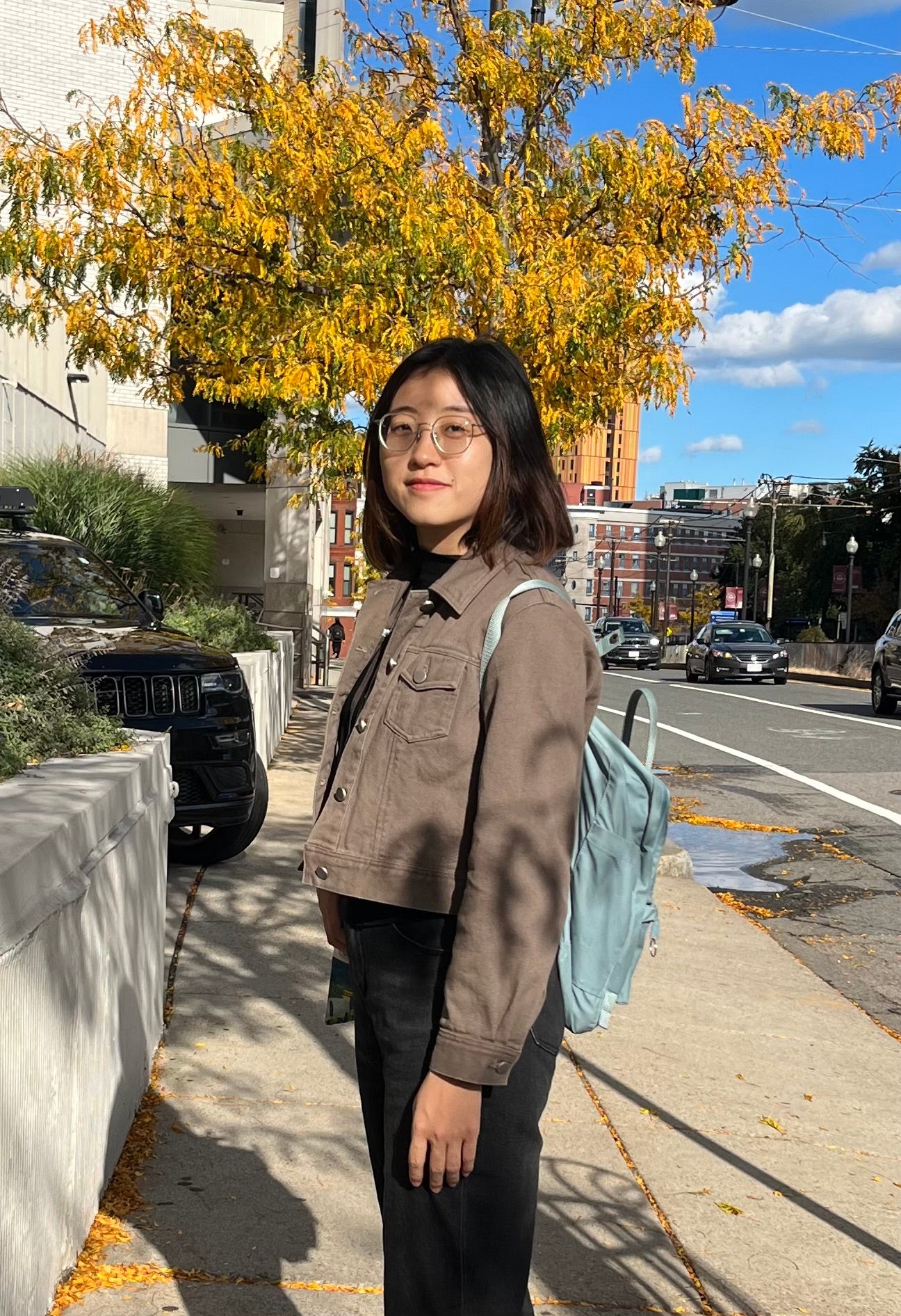
Dr. Yang’s primary focus is to build up robust and statistically efficient methods for causal association discovery, such as large-scale mediation testing and treatment effect estimation problems in complex situations. She is intrigued by the plasma protein data analysis and trying to establish a model for personalized disease prediction.Â
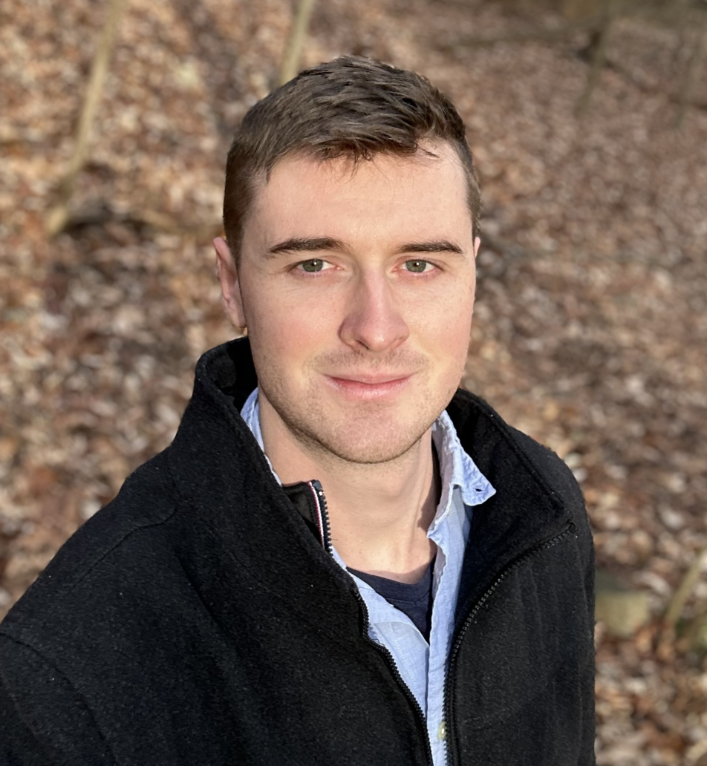
Dr. Barry is working on enhancing the robustness of negative binomial regression methods and quantifying the off-target editing activity of CRISPR systems.
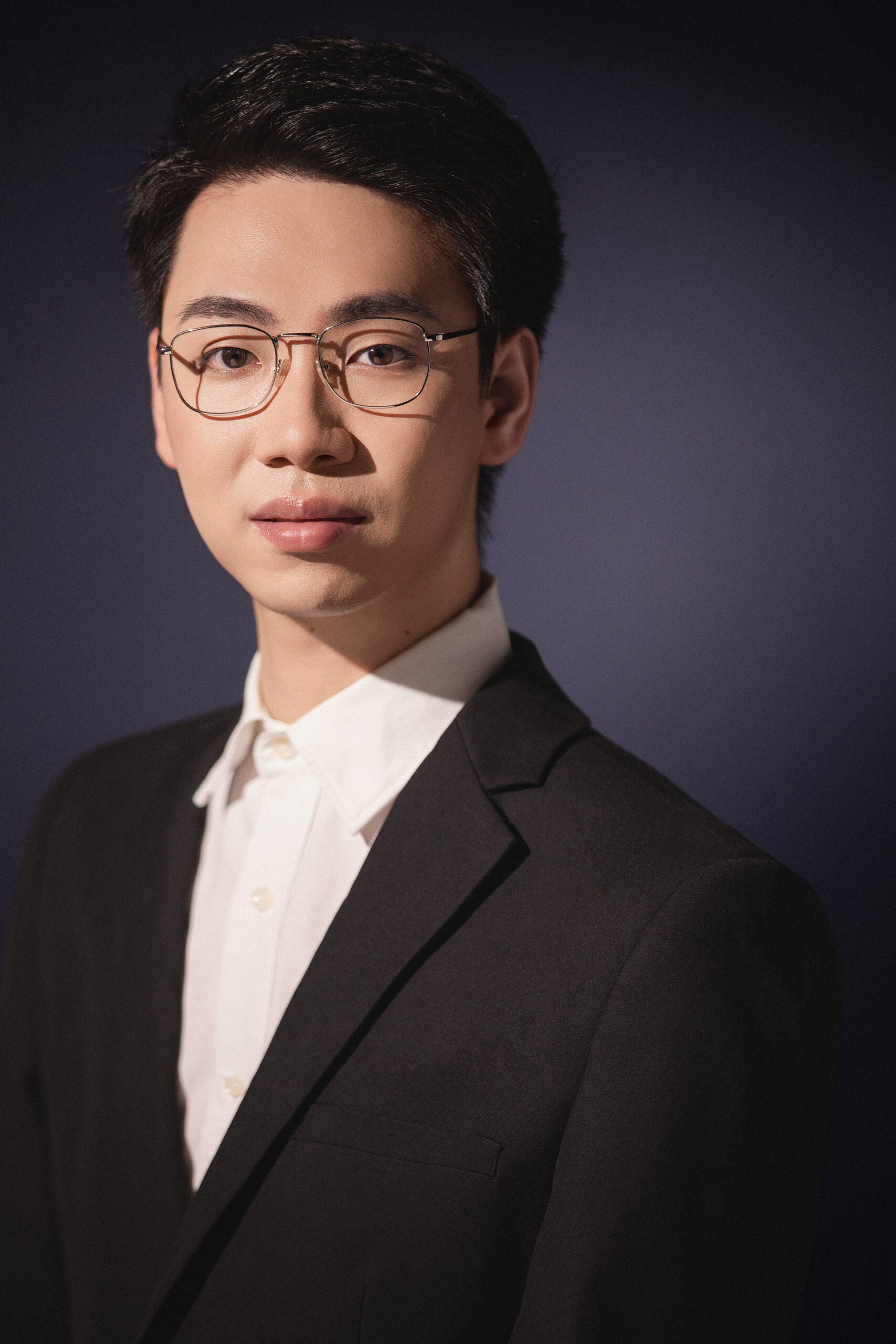
Dr. Chen specializes in developing methods for causal inference and machine learning applied to high-dimensional observational data, with a focus on oncology. His work leverages electronic health records and Flatiron Health data to address challenges in confounding and selection bias through statistical matching, instrumental variable estimation, causal mediation, and sensitivity analysis, advancing robust causal insights in real-world research.
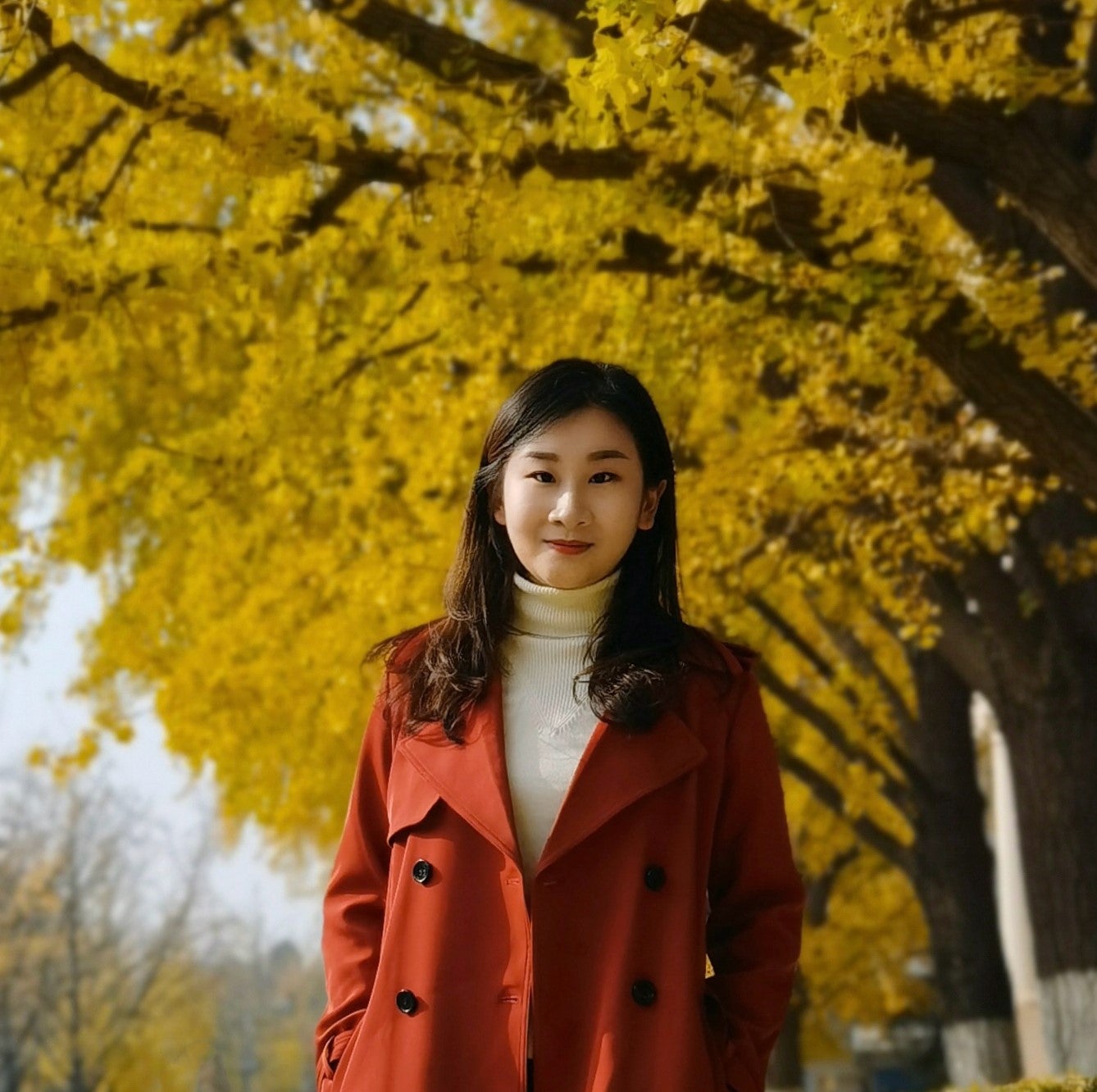
Dr. Song’s research interests include statistical genetics and genomics, Bayesian methodology, and machine learning. Her current work centers on developing statistical tools for large-scale whole-genome sequencing studies, with a particular focus on time-to-event data analysis, polygenic risk prediction, and heritability estimation.
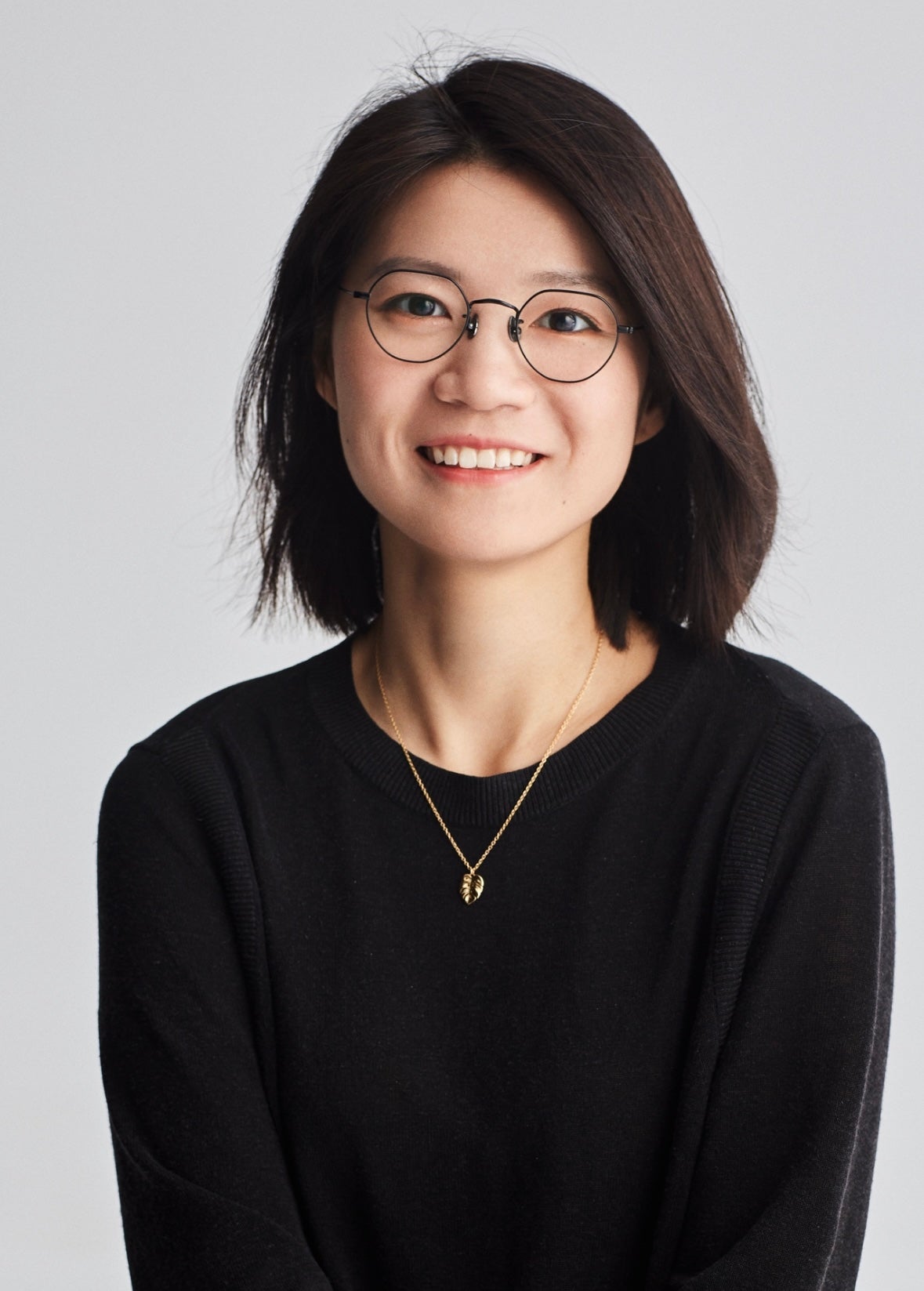
Dr. Wang’s research leverages multi-omics data to understand the molecular heterogeneity of lung cancer and its interactions with environmental risk factors, with goals to elucidate disease etiology and optimize personalized treatment strategies and patient outcomes.
Students
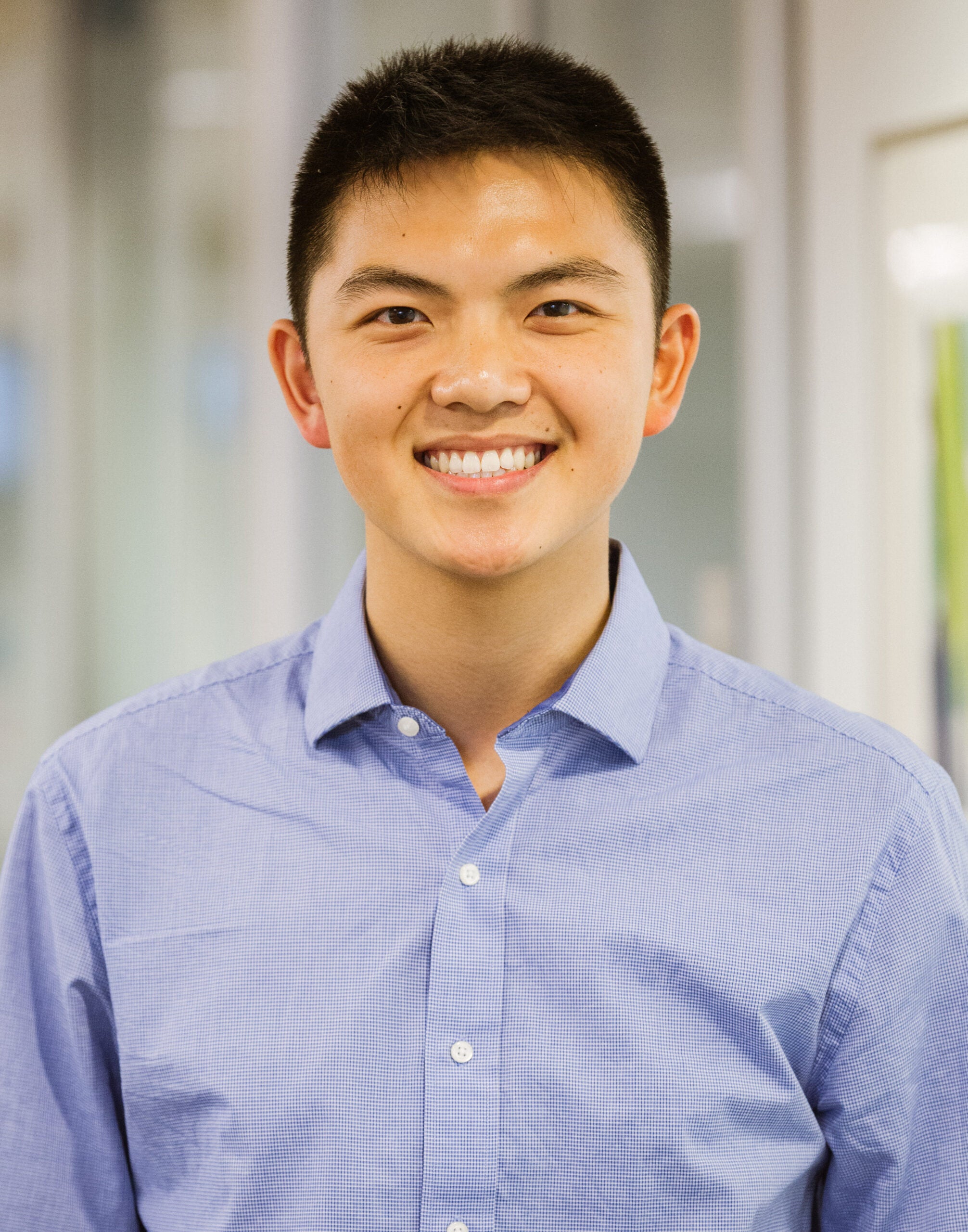
Tony is a 5th year PhD student in Biostatistics. His dissertation research focuses on developing statistical methods to compute polygenic risk scores (PRS) from large-scale biobanks and genome-wide association studies. He also collaborates with the International Lung Cancer Consortium to implement PRS within primary care.
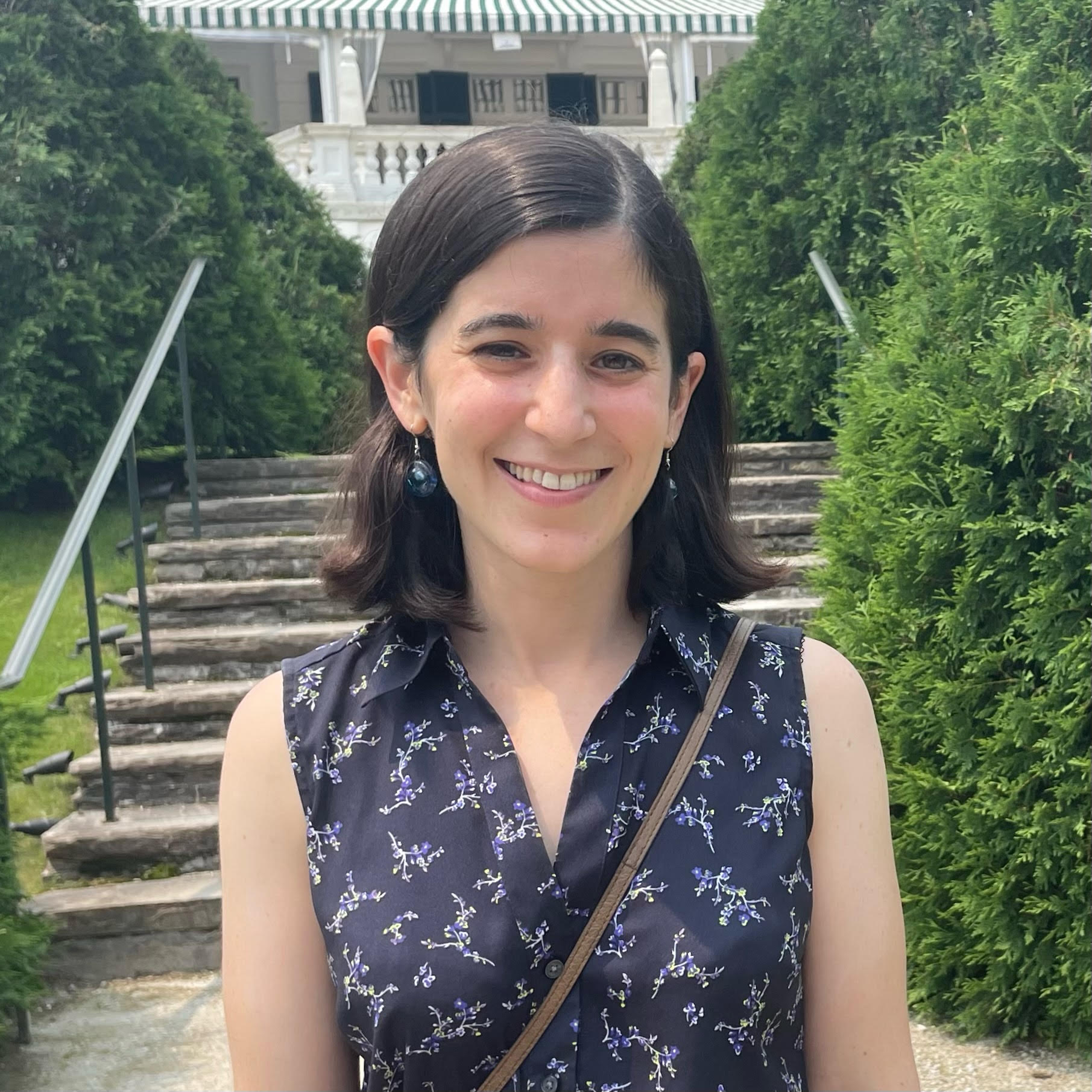
Rebecca is a 4th-year PhD student developing statistical and machine learning algorithms for uncovering latent features in phenotypic and single cell data.
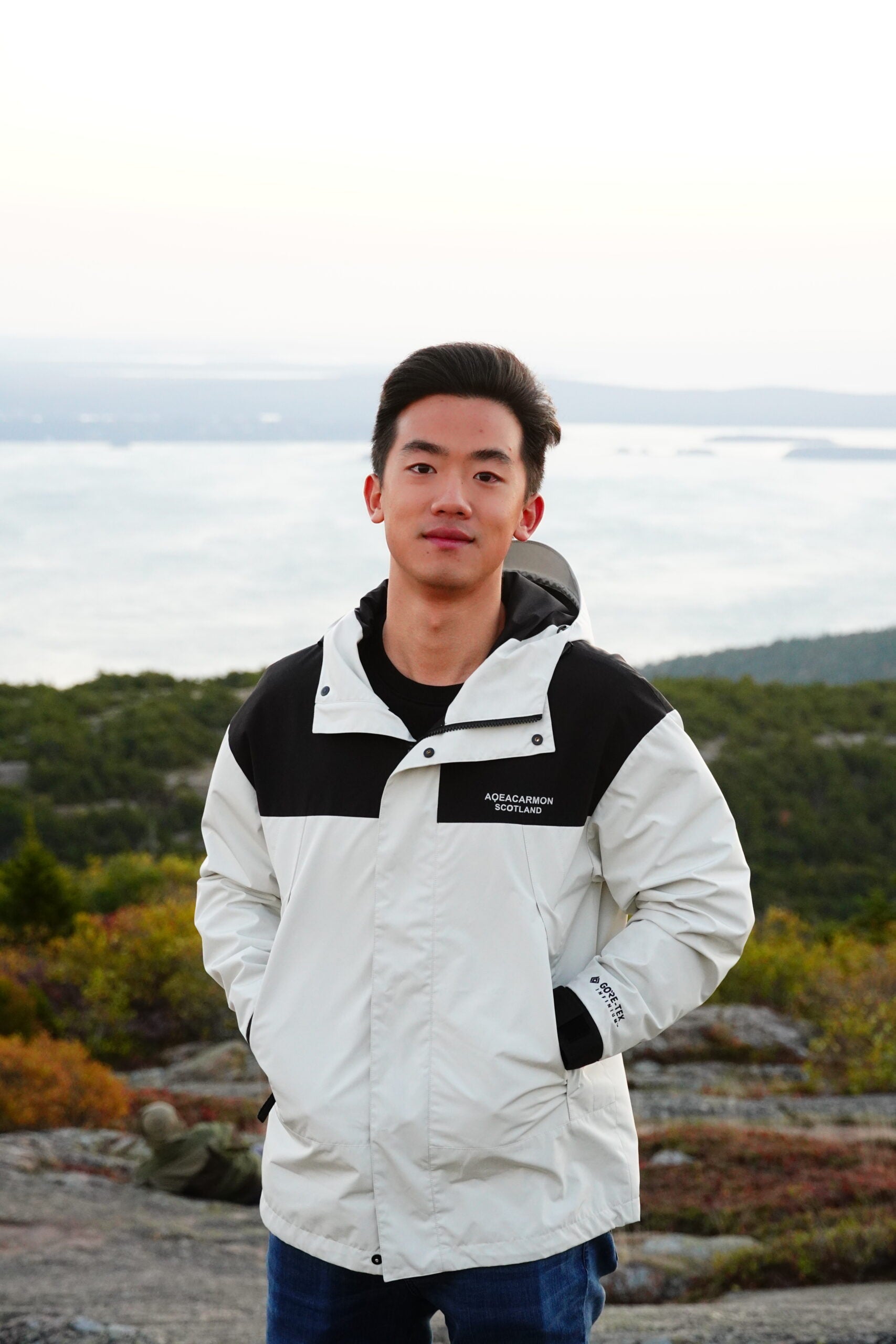
Yuzhou’s research focuses on advancing modern causal inference methods to analyze complex observational studies, with a particular emphasis on developing causal mediation analysis methods for integrative analyses of health outcome data.
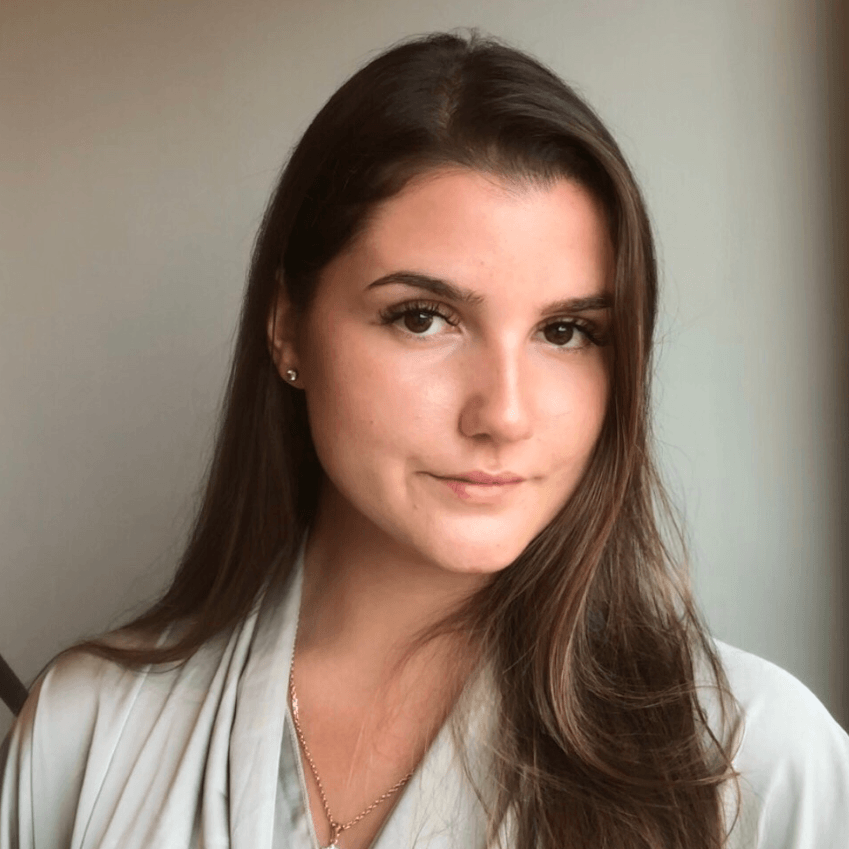
Julie-Alexia’s research focuses on improving statistical genetics methods (such as GWAS and PRS) in multi-ancestry cohorts. She is interested in leveraging differences in genetic architectures between ancestries to discover novel genetic signals and enhancing the inclusion of underrepresented subjects to allow greater generalizability of such findings.
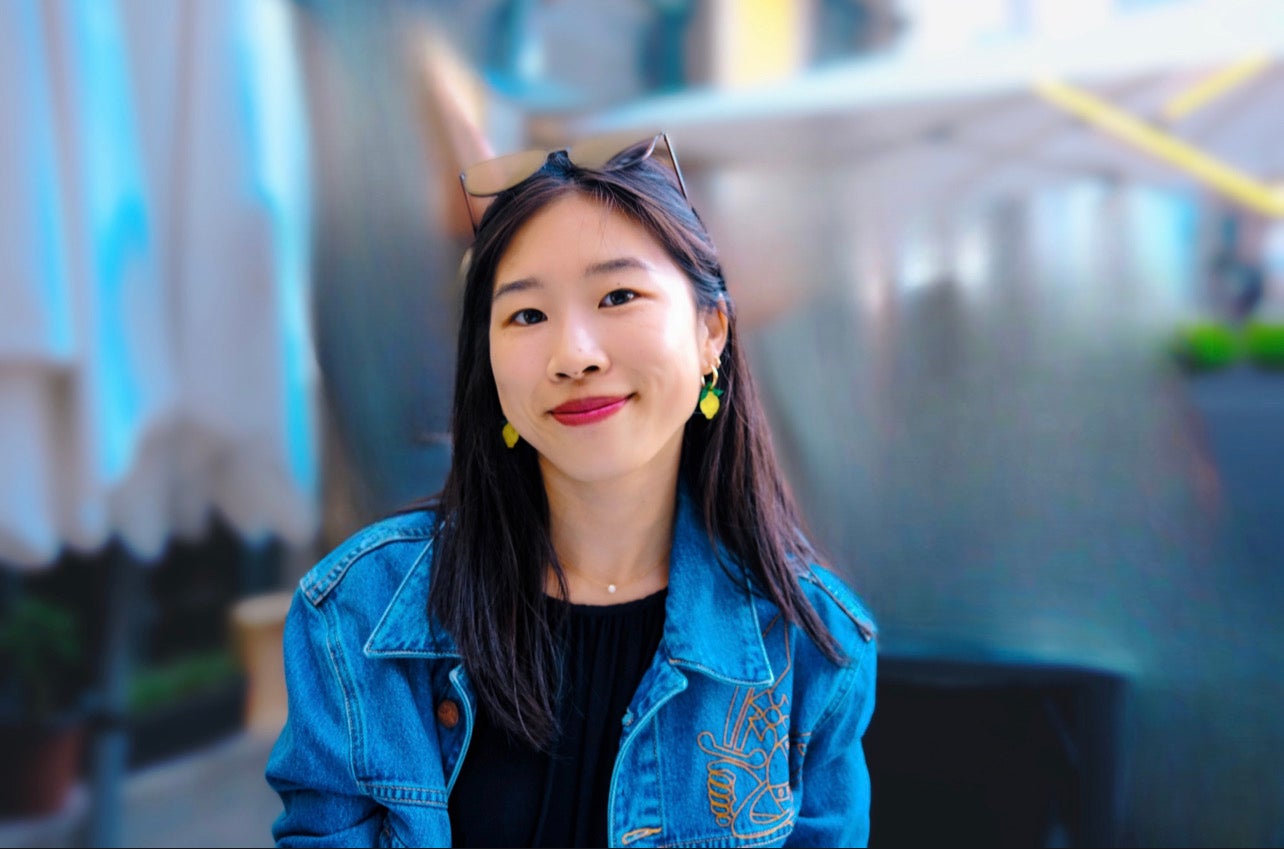
Xiaonan is interested in studying disease mechanisms and identifying drug targets. In particular, she is working on predicting individual-level gene expression to uncover how genetic variants impact phenotype through gene expression.
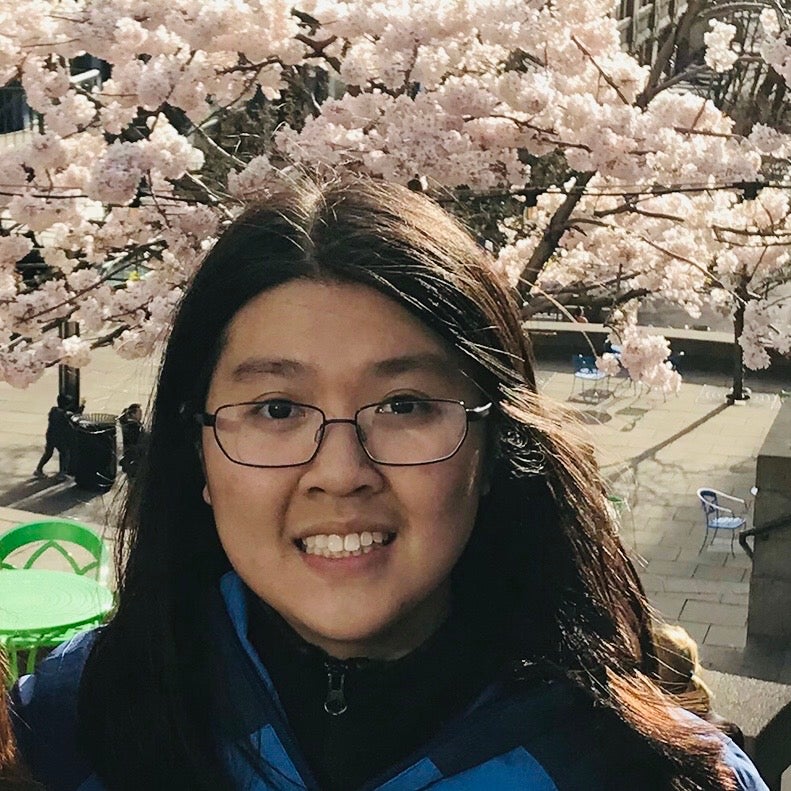
Ziqi is broadly interested in applying spectral methods, high-dimensional statistical inference, and geometrical/topological manifold learning to multimodal genomics and genetics data. Currently, Ziqi is focused on developing computational methods for the IGVF single-cell multiomic data integration and regulatory network inference.
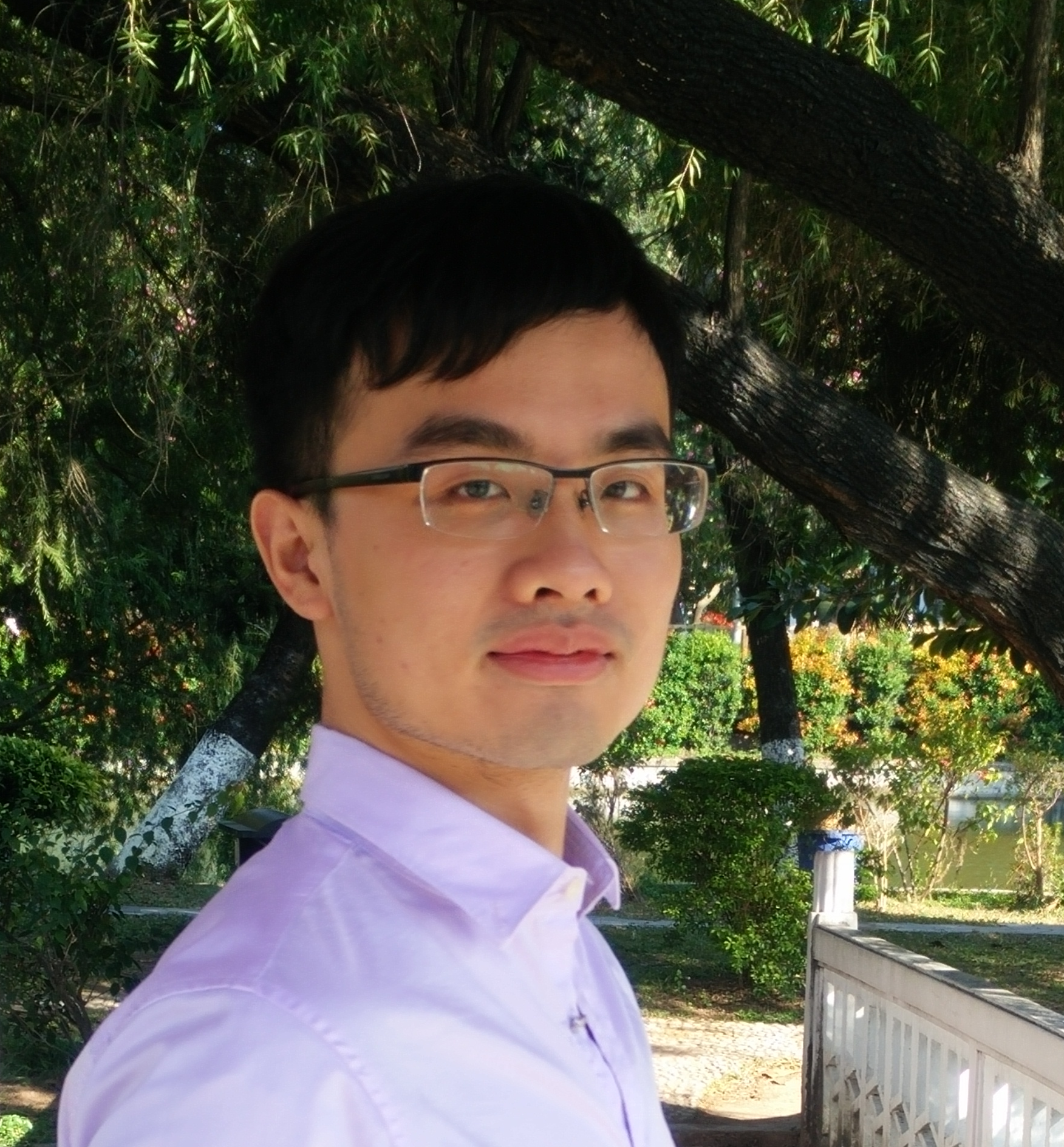
Roman is interested in developing statistical methods to enhance understanding of disease mechanisms and improve patient outcomes, with a focus on integrating genetic and clinical information from diverse sources while accounting for data heterogeneity.
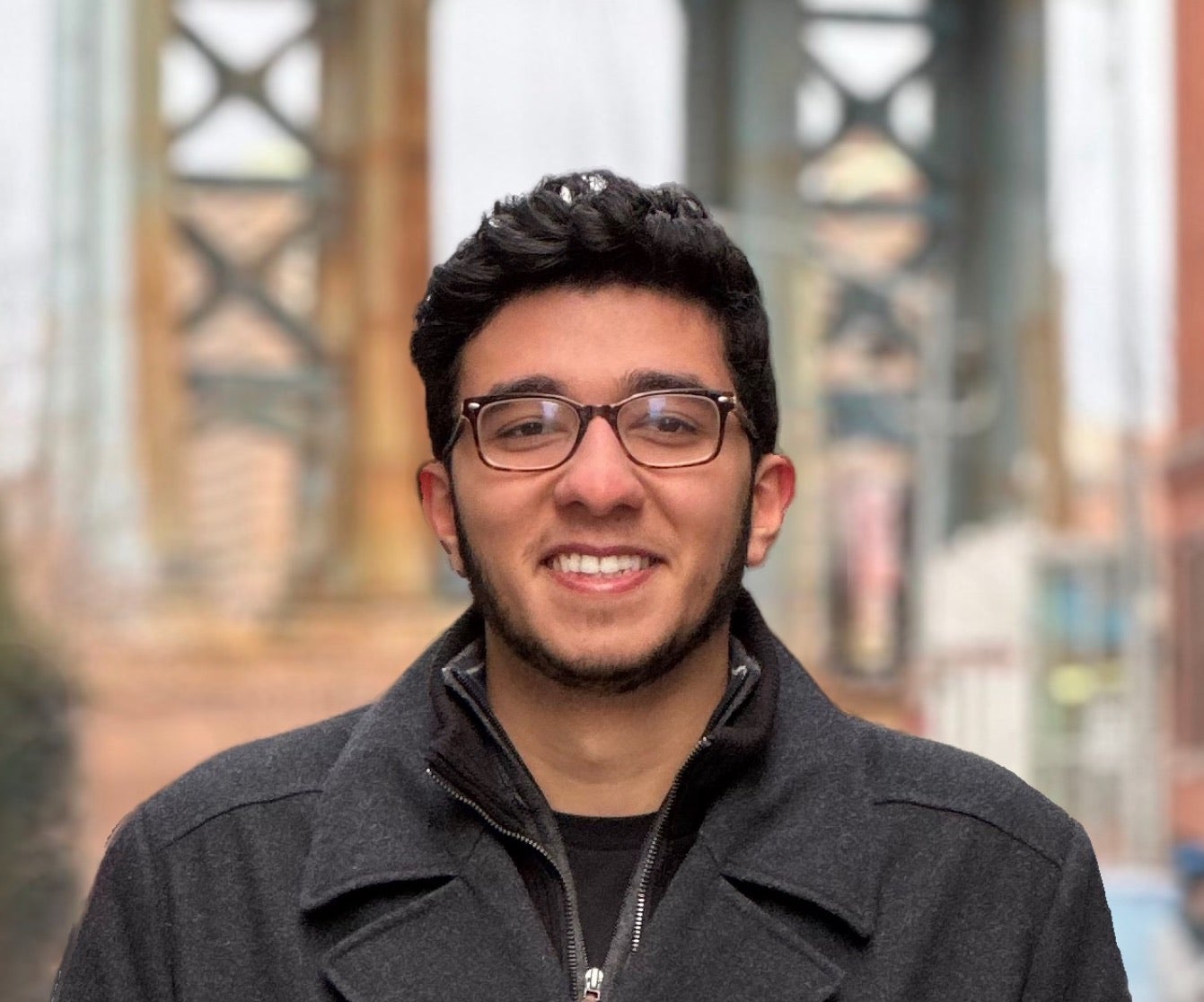