Harnessing AI to model infectious disease epidemics
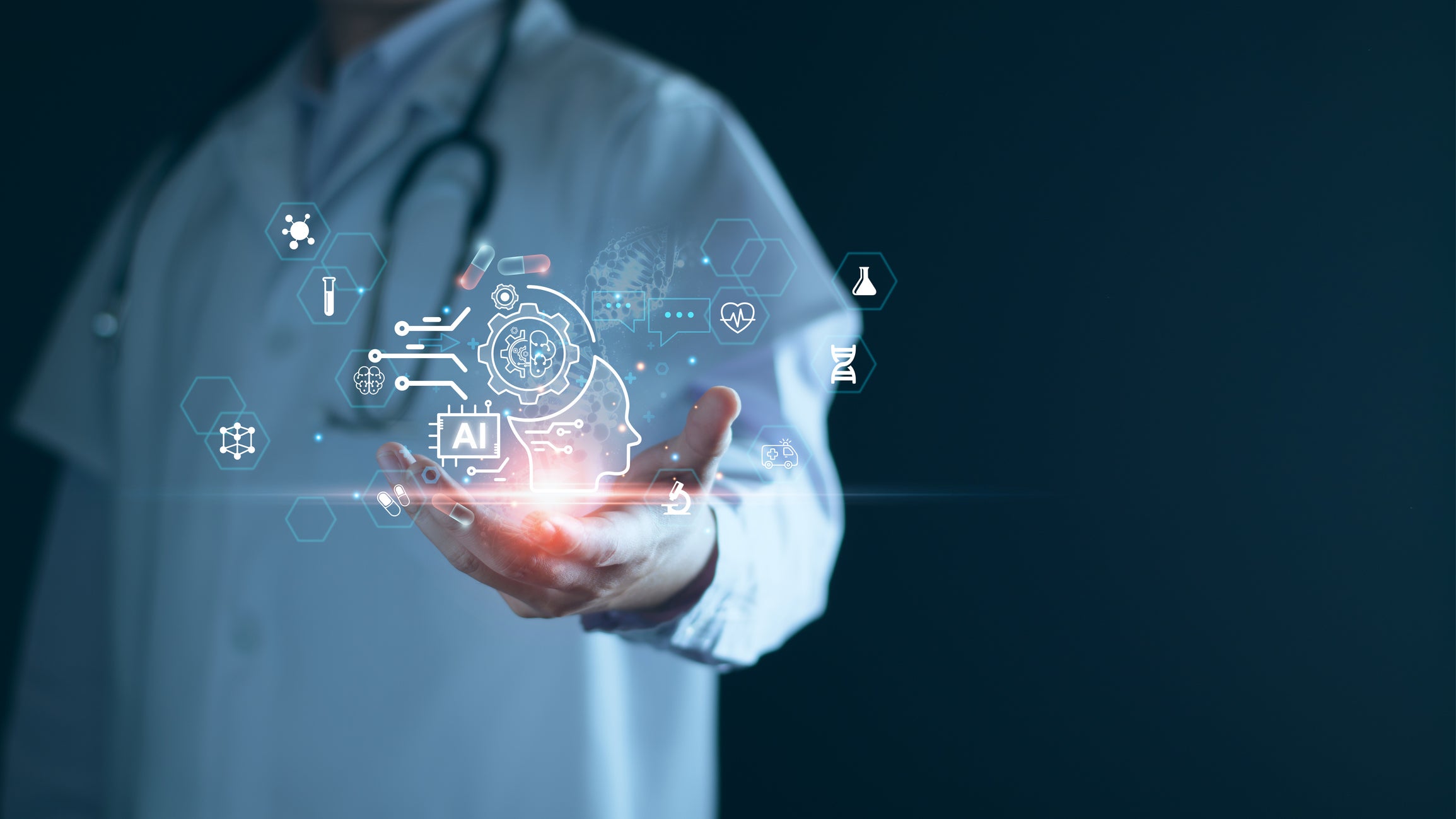
Francesca Dominici, Clarence James Gamble Professor of Biostatistics, Population, and Data Science at Harvard T.H. Chan School of Public Health and faculty director of the Harvard Data Science Initiative, and her research team are developing artificial intelligence (AI) and machine learning models to aid their work on increasing people’s resilience to health threats from environmental stressors and extreme weather events. They are also studying the carbon footprint of AI and developing approaches for responsible and sustainable deployment of AI. She recently spoke about a paper she co-authored in Nature that explored how similar methods could inform decision-making during an infectious disease outbreak.
Q: What are the challenges that arise around infectious disease modeling during an outbreak, and how can AI help?
A: One of the key challenges at the start of an epidemic, and indeed as the infection waves progress, is answering questions about the severity and transmissibility of the infectious pathogen.
In traditional epidemiological analysis, some of these questions can be answered from tightly controlled studies. However, despite major efforts to document what is happening during an outbreak using, for example, contact tracing, idiosyncrasies of the data ensure that the true epidemic process is imperfectly observed.
The actual chain of infection events and where they occur is often ambiguous—individuals may visit multiple locations and meet different people, some of whom might be infectious but do not yet show symptoms—making it challenging to directly measure quantities such as the incubation period or transmission intensity from observational data alone.
AI can accelerate breakthroughs in answering key epidemiological questions via data processing and analysis, speed and efficiency, improved accuracy, and integration of multiple data sources—such as health records, real-time surveillance data, and environmental factors—to create more comprehensive and accurate epidemic forecasts.

Q: What role does data quality and availability play in the effectiveness of AI-driven epidemic models?
A: Data quality is key—we must train AI models with representative data that can capture the features of infectious diseases. The good news is that new AI approaches can increasingly perform well with limited data. Achieving state-of-the-art performance no longer requires months of initial training or terabytes of data.
Assessing human behavior during an outbreak is hard, but AI models can easily account for it if we have the data. For example, data on people’s movements have been extensively used and analyzed in the context of COVID-19 as has data on willingness to vaccinate, use masks, and avoid gatherings. AI can quickly learn and account for the complexity of these interacting processes.
Q: What ethical questions should be considered when using AI to inform infectious disease prevention and control efforts?
A: One set of questions concerns the importance of AI tools being shared equitably for use by public health authorities. The effectiveness of this will depend on the development and sharing of expertise within collaborative approaches to surveillance and analysis.
A second set of questions concerns how AI tools will be deployed in the design and implementation of public health policy. An important lesson from COVID-19 was that all policy decisions are at their core value judgments with a strong ethical component, for example, about the distribution of vaccines or the limits of privacy and liberty in the use of digital contact tracing. Such judgments must be subject to deliberation and be justified and accountable.
In the paper, we talk about how novel methodologies from AI can improve the collection and merging of key data and how they are included in decision-making frameworks to improve population health. These advances must also be equitable to avoid deepening health inequalities.
Demonstrating the effectiveness of AI in improving policy decisions that benefit population health remains one of the biggest challenges. For AI to be successful in that regard, the coming years will see a growing need for close collaboration among researchers, policymakers, and society.