Artificial intelligence’s potential health benefits, risks discussed at conference
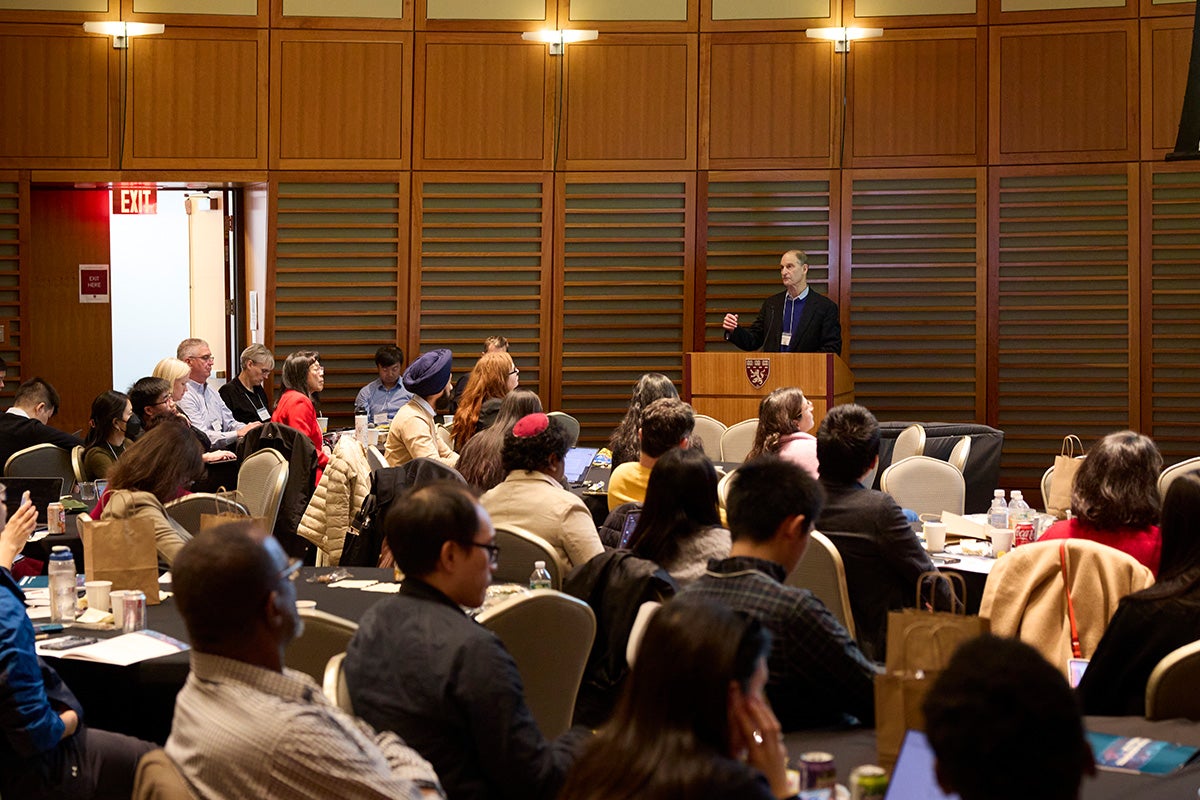
October 23, 2024 – This year marked a milestone for artificial intelligence (AI)—for the first time, the technology’s importance was recognized with a Nobel prize.
The 2024 Nobel Prize in Chemistry was awarded to researchers who developed software including AlphaFold, an AI model that predicts the three-dimensional structure of proteins. The model can be applied to a broad range of fields, including health—for example, it could help researchers to better understand viral proteins, leading to improved vaccine design.
AlphaFold was just one of many AI technologies discussed at the 18th annual conference of the Program in Quantitative Genomics at Harvard T.H. Chan School of Public Health. Held October 17–18 at the Joseph B. Martin Conference Center, the event featured presentations, a panel discussion, and poster session where researchers addressed both the potential benefits and risks of using AI to advance health.
Applying AI to diverse diseases
One application of AI is using it to tackle the issue of antibiotic-resistant bacterial infections. In recent decades, the number of resistant bacterial strains has increased, yet the number of new antibiotics discovered and approved by the U.S. Food and Drug Administration (FDA) has been decreasing, according to James Collins, the Termeer Professor of Medical Engineering and Science at the Massachusetts Institute of Technology, one of the keynote speakers.
“The golden age of discovery of antibiotics was in the ’40s, ’50s, and ’60s—before the molecular biology revolution, the genomics revolution, the AI revolution, the biotech revolution,” he said. “We’ve had a discovery void in these last few decades.”
Collins is developing various AI models to identify potential new antibiotic molecules. In a 2020 study, he and his colleagues took a collection of thousands of drug molecules that had been approved by the FDA—to treat any disease, not just infections—and tested them in the lab to see whether they prevented bacterial growth. They trained an AI model based on the results, and then used it to predict the potential antibacterial activity of a new set of molecules. The approach uncovered a promising molecule with a novel biological mechanism that, when tested in mice, successfully lowered the growth of multiple types of antibiotic-resistant bacteria. Collins is now exploring ways to bring the molecule to the clinic.
The researchers have since applied the AI method to different bacteria and larger collections of molecules. “This work came out … right as the world was getting anxious about AI,” Collins said. “And interestingly, this got elevated [by AI supporters] as an example of AI for good—[they said] don’t regulate it too much, because you want to be able to get after new molecules to go after these pathogens.”
AI also has applications in improving cancer treatment, according to Olivier Elemento, a professor of physiology and biophysics at Weill Cornell Medicine in New York City. He has developed AI models that predict which cancer drugs may be effective for an individual patient, based on their unique genomic sequence, gene expression patterns, or other factors.
“We could use data from thousands of patients and more, and we need to be able to integrate pretty complex information,” he said. “We can’t do that without using tools like AI.”
Advances in generative AI
Multiple conference speakers discussed the health applications of generative AI technology, which includes chatbots such as ChatGPT. In contrast to Collins’ and Elemento’s AI models, which use specific data to generate predictions, generative AI is trained on vast amounts of data to respond to any number of questions a person might ask.
Shekoofeh Azizi, a staff research scientist at Google DeepMind, shared her work in developing generative AI models that help clinicians perform a wide variety of tasks, from analyzing a description of patient symptoms and providing a diagnosis, to looking at an X-ray image and writing a report about what it shows. The models integrate multiple types of data—such as medical image findings, electronic health records, lab test results, and genomic information—in order to produce results that may not be possible using a single type of data.
“We are in the very earliest stage of actually understanding the opportunity that we have,” Azizi said.
Regulatory challenges
Using generative AI in the clinic poses a host of different risks, according to keynote speaker David Blumenthal, professor of the practice of public health and health policy at Harvard Chan School. The models may respond incorrectly to questions, change answers when asked the same question at different times, or provide results that are biased against underrepresented patient groups.
Some government regulations for the responsible use of AI have been recently implemented. A U.S. presidential executive order announced in 2023 contained a range of provisions, like directing federal agencies to develop standards for AI safety, fraud protection, and data privacy. The European Union (EU) AI Act, which went into effect August 2024, bans high-risk AI uses, such as manipulating people to change their behavior, and requires generative AI developers to provide comprehensive technical documentation. However, those regulations may not be sufficient, particularly for generative AI in health, Blumenthal said.
“Nobody has a clue about what to do about generative AI. The FDA doesn’t, the EU doesn’t—they grapple with it, and they kick it down the road,” he said. “In the meantime, clinicians are using it for patient care.”
Because the applications of generative AI models are so broad, assessing their performance is difficult, Blumenthal said. He proposed a new regulatory approach based on how clinicians are currently evaluated. Just as clinicians must complete certain training and pass licensing exams, the government could set similar requirements for generative AI, such as standardized training material and tests.
Another speaker—Hoda Heidari, the K&L Gates Career Development Assistant Professor in Ethics and Computational Technologies at Carnegie Mellon University—noted that for generative AI, evaluating models only based on technical accuracy is not adequate because of the open-ended and uncertain ways that clinicians might interact with them. She recommended that rather than building AI models and then figuring out how they can be applied in the clinic, clinicians should be included in the development process from the beginning. That way, researchers can determine whether the technology actually addresses a real need.
“It’s important for us to keep in mind that in practice, AI is really an immature technology,” she said. “While we have made good progress in the past couple of years to move towards a more robust and dependable system of governance, we are nowhere near that system yet.”
– Jay Lau
Photo: Kent Dayton