Data science controversies explored at Zelen Symposium
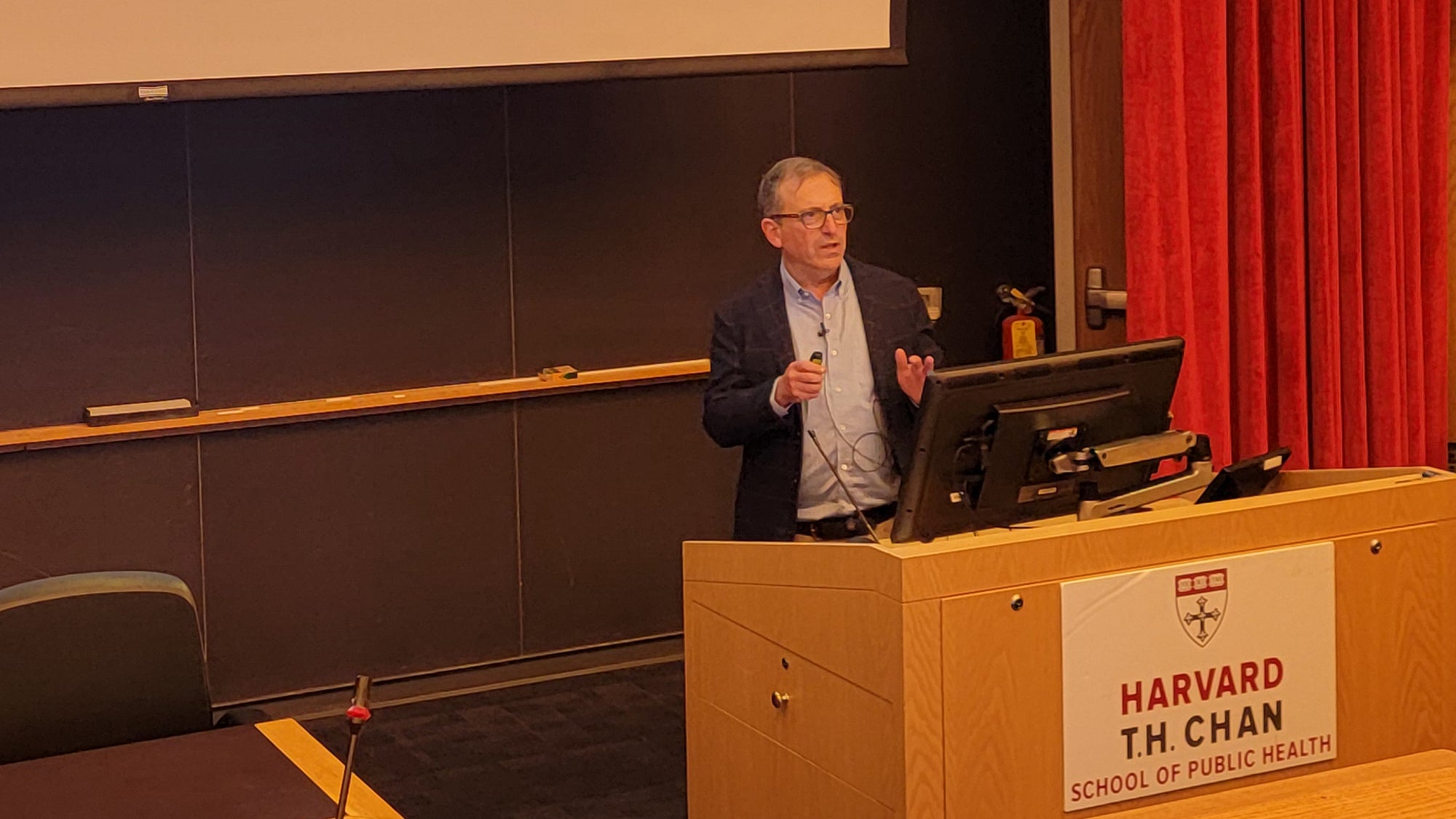
During the early stages of the COVID-19 pandemic, vaccines and treatments had not been developed yet—so public health officials had to rely on nonpharmaceutical interventions (NPIs) to stave off infections. One intervention was contact tracing, which involved identifying individuals who may have been exposed to an infected person, so that they could isolate and prevent further disease spread.
But there was a problem—researchers couldn’t test whether contact tracing was actually effective, because it would have been unethical to set up an experiment that deliberately stopped contact tracing for comparison purposes.
Then something unexpected happened: Public health officials in England announced that due to a technical error, contact tracing data for part of the population had not been properly recorded for a week. “They got a beautiful natural experiment by not tracing a third of the contacts,” said Marc Lipsitch, professor of epidemiology at Harvard T.H. Chan School of Public Health, speaking at the annual Marvin Zelen Memorial Symposium. Data analysis showed that contact tracing indeed led to fewer infections, Lipsitch said. He added, though, that debate continues on the effectiveness of other types of NPIs for COVID-19.
The symposium, held April 4 at Harvard Chan School, featured experts who discussed controversies in the field of data science, including the pros and cons of using artificial intelligence (AI) in medicine. The event was organized by the Department of Data Science at the Dana-Farber Cancer Institute in memory of its former chair Marvin Zelen, who also served as chair of Harvard Chan School’s Department of Biostatistics.
Impacts of NPIs during COVID-19
During his talk, Lipsitch compared different studies about NPI effectiveness that came to opposing conclusions.
“My argument is that there is theory, history, and data on the short-term effects of nonpharmaceutical interventions suggesting that they prevent cases and deaths in epidemics,” he said. In addition to the natural experiment on contact tracing, he cited a study about the effect of large group gatherings on COVID-19 cases. Researchers found that in England, whenever a soccer game was aired on television—leading people to gather together to watch—a spike in cases occurred.
On the other hand, other studies have shown that NPIs such as mask mandates and business closures did not have any impact on COVID-19 cases. Lipsitch noted several flaws in the studies’ methodologies, including failing to account for the possibility of reverse causality—instead of NPIs influencing the number of COVID-19 cases, the relationship could actually be the other way around. For instance, a surge of cases could have led public health officials to implement NPI policies, while a decrease in cases could have led them to relax policies.
Using AI in medicine
Sayash Kapoor, Ph.D. candidate at the Center for Information Technology Policy at Princeton University, discussed the potential pitfalls of applying AI technologies to medicine.
“We have been seeing this drastic uptake [of AI and machine learning] across scientific fields, and in my opinion, this is cause for alarm. There are many risks inherent to the adoption of AI,” he said.
Kapoor shared examples of AI models that were developed with incorrect methods, such as models that aimed to predict whether an individual had COVID-19 based on X-ray or CT scan images of their lungs. In several different studies, researchers collected images from some individuals with COVID-19 and some without, then trained the models to recognize the differences and make predictions—but the images chosen were biased, which researchers did not realize would affect the results. Individuals with the disease were all adults, while those without were all children—so the models ended up recognizing differences due to age rather than infection status.
“Errors … take a long time both to uncover and to consequently challenge, and in the meantime, there’s this false scientific consensus that’s created around the applicability of machine learning,” Kapoor said. To improve AI models, he proposed a checklist for researchers that lays out best practices for each step of model development, from choosing the right data to evaluating the model correctly.
Other symposium speakers included Arjun Manrai, assistant professor of biomedical informatics at Harvard Medical School, who spoke about the successes and challenges of using AI in medicine; Alina Chan, a former scientific advisor at the Stanley Center for Psychiatric Research at the Broad Institute, who presented evidence suggesting that the COVID-19 virus originated from a lab; and Gavin Schmidt, director of the Goddard Institute for Space Studies at the National Aeronautics and Space Administration, and Auroop Ganguly, College of Engineering Distinguished Professor at Northeastern University, who discussed the biases and challenges of climate change computational models.