From Data to Local Action: AI-Powered Analytics for Crisis Preparedness
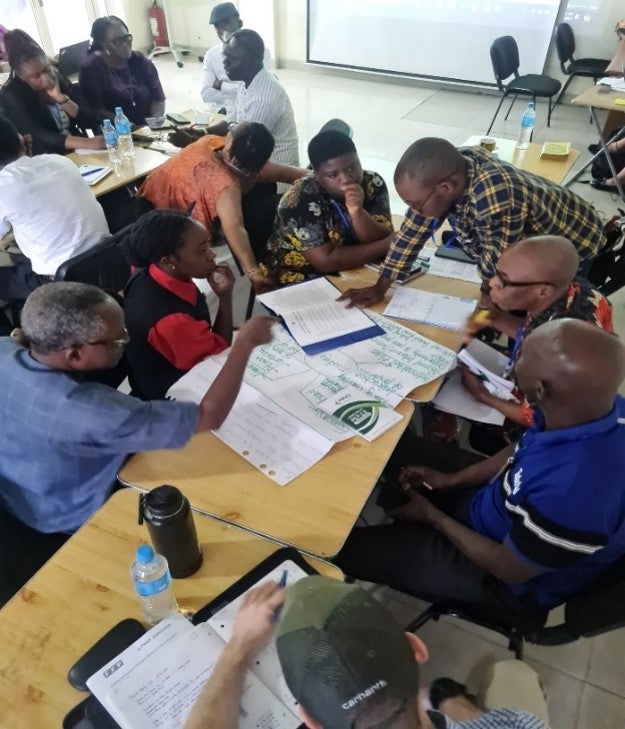
To state the obvious: no crisis exists in a vacuum. Whether it begins with a pandemic, a natural disaster, or political unrest, the ripple effects inevitably reach across social, economic, political, and security systems. A health crisis, for example, is never just a health crisis. And the same goes for any emergency. The hard truth is that preparedness means being ready to act on multiple fronts at once—everywhere, with limited resources, and diminishing influence as events escalate. This is where advanced analytics and generative AI come in.
It is much harder to predict the cascading impacts of the crisis after it occurs.
Predictive models are of little use if all they do is tell you the location where a particular event will occur. That’s the easy part, whether through regressions, time series, cluster analysis, event history, geospatial methods, Bayesian analysis, AutoRegressive Integrated Moving Averages (ARIMA), or any combination of these and other approaches. It is easy to determine which countries are most susceptible to fires, floods, earthquakes, or terrorist attacks. It is harder to predict the timing and severity. It is much harder to predict the cascading impacts of the crisis after it occurs.
But policy makers need to prioritize response options somehow, often without a solid empirical basis to guide them. As a result they tend to 1) default to precedent (“fighting the last war”), 2) defer to influence-based decision making (deference to the “loudest voice in the room”), or 3) simply react (playing “whack-a-mole”), reluctant to allocate scarce resources on things that are not already escalating.
To address this challenge, Fund for Peace has partnered with SAS to develop a Crisis Sensitivity Simulator, to help decision-makers and responders prioritize the use of their limited resources to maximum effect. The simulator does not predict the onset of a crisis, but it takes nineteen years of historical Fragile States Index data for 179 countries, to determine how a given crisis is likely to unfold in a particular country, if and when the shock occurs.
The tool employes a three-step process as follows:
Step 1. The first step of the process uses a 4-parameter beta distribution to model country-specific factor behavior under normal conditions. It then applies shocks to individual factors, runs multiple simulations, and visualizes the results through box plots, comparing baseline and shocked distributions to assess a country’s sensitivity to different types of crises.
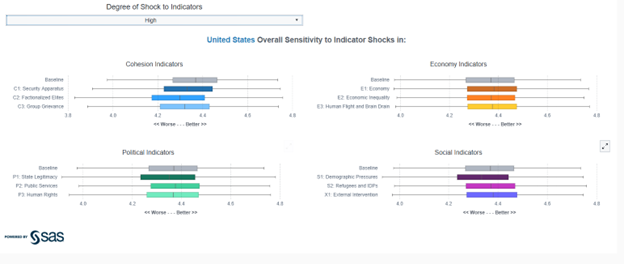
Step 2. Then the tool analyzes the correlations between different types of shocks over time in each individual country in order to help subject matter experts infer how a given crisis may cascade across different sectors.
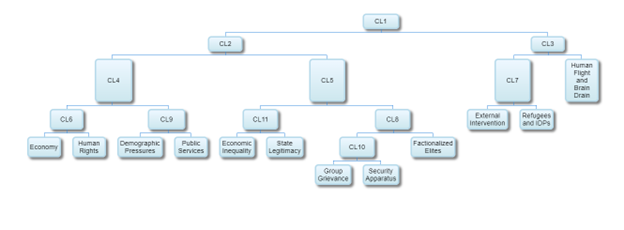
Step 3. Finally, the tool integrates these analytics into a customized prompt generator, which leverages a Large Language Model (LLM) to produce detailed, localized crisis scenarios. This process accounts for both current conditions and historical trends, generating nuanced narratives that illustrate how a crisis may unfold across different communities. By incorporating data-driven insights with natural language generation, the simulator helps decision-makers anticipate cascading impacts, assess vulnerabilities, and craft more targeted response strategies. This tool showcases how responsible and trustworthy AI can optimize decision-making in complex humanitarian scenarios.
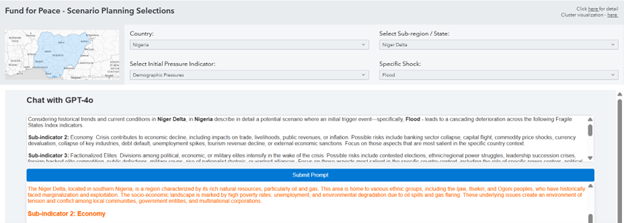
Importantly, this tool is not a replacement for local knowledge.
Importantly, this tool is not a replacement for local knowledge. Rather it is used for brainstorming and “red teaming”: helping teams explore a range of potential outcomes and cascading effects and facilitating alternative analysis by challenging assumptions and considering different perspectives. However, when we tested it in Port Harcourt, Nigeria, for a crisis scenario planning exercise on catastrophic flooding with humanitarian, peace, and development practitioners, the participants found the analytics and the AI enhancements useful for validating and amplifying their own foundation of expertise that they brought to the question for prioritizing strategic preparedness and response.
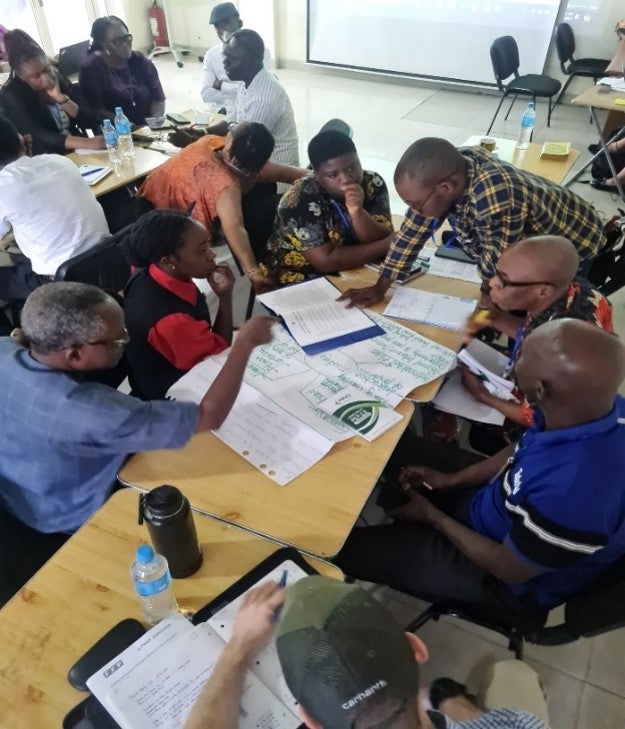
In May, we will be presenting the tool at SAS Innovate 2025, which is an annual conference focusing on advancements in data analytics and artificial intelligence that is attended by tech luminaries sharing their latest breakthrough ideas and projects. However, the real potential of the tool is not found supporting the intelligentsia, but that it makes advanced analytics accessible to local experts and practitioners in the field so that they can prioritize and target their preventative efforts and limited resources for maximum effect, in a world where the intensity and frequency of global shocks are increasing. This is all the more important at a time when guardrails and stabilizing mechanisms like international norms, multilateral cooperation, and evidence-based policymaking are being tossed aside by the most powerful country in the world.
Nate Haken is an expert in early warning and conflict sensitivity and a member of the APL Steering Committee.