How Health Care Leaders Can Build Effective, Bias free AI
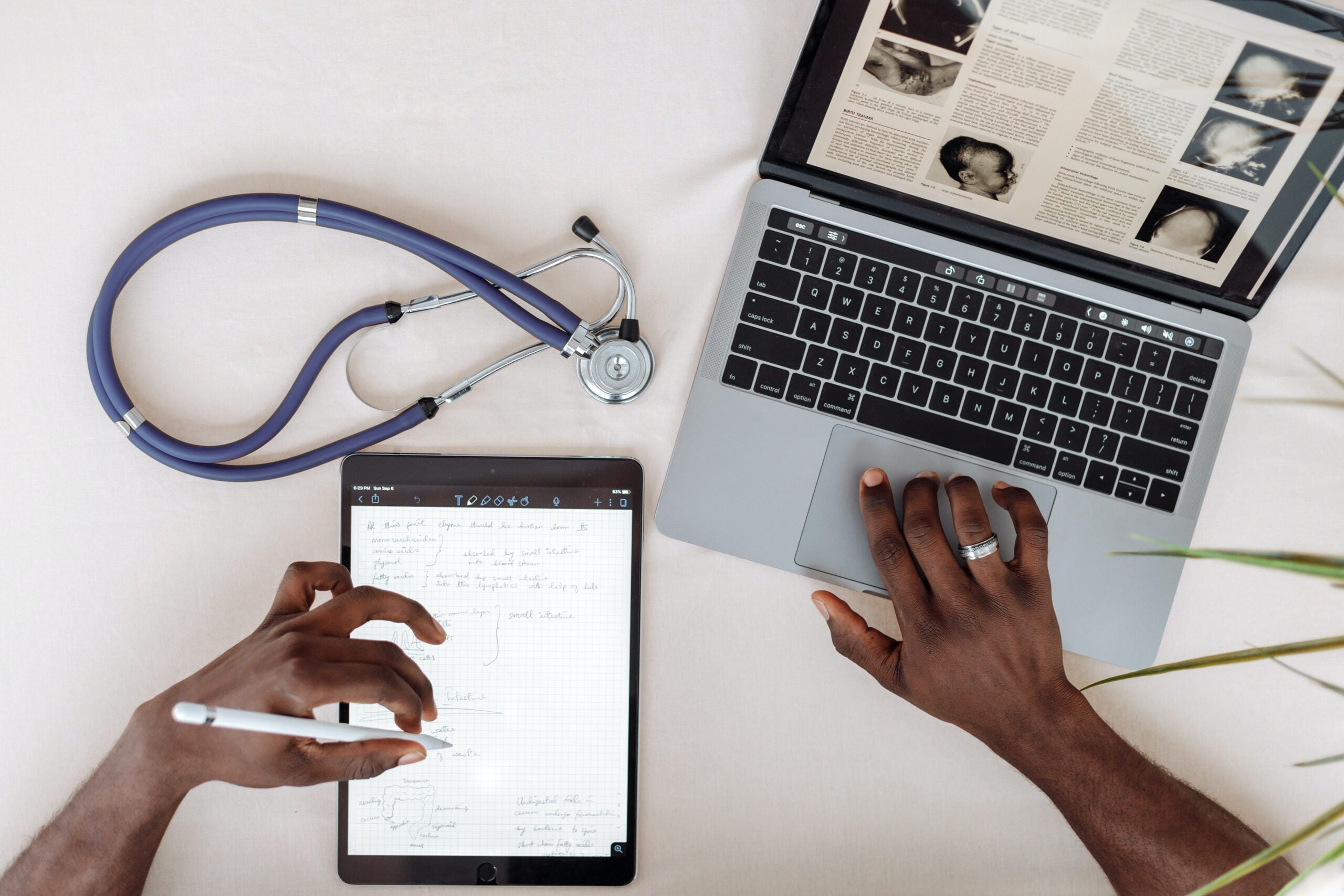
As advances in technology have continued to evolve, many health care leaders and clinicians have been exploring the promise of artificial intelligence (AI) to empower patients and practitioners and improve the efficiency of health care organizations. Some of the benefits these technologies can bring to health care range from the ability to predict who is at risk for diseases, to using data to improve diagnostic processes, to learning from different use cases which treatments will yield the most effective outcomes for different patient groups.
Yet in reality, even though AI is now deeply embedded in the cultural consciousness, there is limited evidence of its real-world impact in medicine outside of research studies, according to Gopal Kotecha, a senior teaching assistant who previously served as Program Director of Harvard T.H. Chan School of Public Health’s Responsible AI for Health Care: Concepts and Applications and Innovation with AI in Health Care programs.
This new certificate program is geared to clinicians and health care leaders who want to better understand the role of AI and learn how to apply it to inform decisions in a meaningful way to improve processes and performance and create more equitable systems.
Challenges Hampering Widespread AI Adoption
“Even as the field of AI has matured in recent years, it hasn’t lived up to earlier predictions of where it would be at this point,” Kotecha says. “We need to take this opportunity to come together in multidisciplinary groups to explore the causes that are hampering its effectiveness and figure out how to address them, in order to make sure we are driving this technology in the right direction,” he adds.
Trishan Panch, MPH, MD, who is an experienced healthcare entrepreneur and also serves as Program Director of Harvard’s AI for Health Care, as well as President of the Alumni Association and an Instructor at the Harvard Chan School, says that a major factor preventing AI from reaching its potential is the lack of awareness regarding best practice in developing and scaling these technologies amongst busy health care executives. “The truth is that most health care leaders don’t have backgrounds in computer science or data science so these technologies seem overwhelming to them,” Panch says. As a result, they steer away from these novel tools since they are worried about incurring risk, harm or unnecessary cost for their organization. “Further complicating matters is that most executive education in this area is either too technical or does not adequately cover important issues such as algorithmic bias, change management, teamwork, regulation or the importance of rigorous evaluation,” he says.
The Challenge of Algorithmic Bias
Heather Mattie, PhD, SM, MS, a Lecturer in Biostatistics at the Harvard Chan School and third Program Director for AI in Health Care, further expanded on one of the principal issues with AI in healthcare – the issue of algorithmic bias. First, disadvantaged populations (including different racial, gender, disease, age and socioeconomic groups) are often underrepresented in research so the numbers don’t tell the full story.
Further compounding the problem is that more vulnerable populations often have less access to health care and to technology than people with higher socioeconomic status, which skews any observational data. Finally, seemingly innocuous design shortcuts when building AI systems can lead to bias-perpetuating prediction models.

The Danger of Not Including Minority Groups in the Data
All of these factors have created a technological system that perpetuates the very inequities it was created to solve, Kotecha stresses. “We had a lot of good intentions for AI that have ended up falling short,” he adds.
Mattie offers the following scenarios to illustrate the dangers that exist with the first generation of AI based tools: Suppose you develop an AI tool to detect skin cancer in patients. Yet the data that is used to train the system to recognize what this cancer looks is all based on predominantly Caucasian skin tones. This means that the AI tool may not be able to detect skin cancer as effectively in people with different skin tones. If such a tool were implemented without appropriate consideration of the risks, this could mean missed cancer or unacceptable outcomes in historically disadvantaged groups.
Panch adds: “Perhaps if you are doing an academic research project and only have access to a single dataset, it is acceptable to build such a one-dimensional tool and just highlight the weaknesses when applying to other skin tones. But by the time you implement this in the realities of the clinic, these considerations should have been addressed a long time ago.”
Panch also highlights recent research that shows how design shortcuts led to a biased health system in practice. A commercially used algorithm that guided health care decisions used health care cost as a proxy for need. Since black patients actually have less health care spending for the same clinical need, the algorithm’s risk allocation was found to assign the same level of risk to sicker black patients than white patients. “In this scenario, the healthcare organization was open enough to collaborate on the research and change the system once a bias-perpetuating algorithm was found,” Panch points out. But he adds that this example raises an important question: “How many other [bias algorithms] are still out there? It will take both a cultural change and knowledge in the community to ensure it doesn’t become pervasive.”
How do Address the Challenges with AI
While the challenges that exist are clear, determining exactly how to address these problems is much more complicated, Mattie says. She stresses that it requires bringing together multi-disciplinary groups to raise awareness about the issues and to begin to change an ailing system.
Mattie, Panch and Kotecha offer the following steps to guide these efforts:
- Become more aware of the problem and help to communicate it more broadly to other people within your organization so they can respond accordingly. For instance, for data scientists, it is essential to be informed about the limitations of the information you are using to base decisions and diagnoses. For health care executives, it’s also important to know what questions to ask to assess the potential—and weaknesses—of AI and the algorithms used so you can keep your expectations realistic.
- Make sure your organization audits their algorithms so everyone is aware of how accurate they are. This requires understanding the source of the data you use, as well as the experiences of the teams that trained the programs to process the data, in order to assess how well your efforts will serve the population you are trying to reach.
- Recognize that some populations will naturally be underrepresented since diseases or ethnic subgroups are just inherently rare. Once you acknowledge what’s missing, be sure to have an honest conversation about the weaknesses and make a good-faith effort to include those populations. Here, working as a community with governments, charities and private enterprises is very important.
- Be creative with how to best address the limitations. It doesn’t mean you can’t use data that doesn’t fully include everyone’s experiences, but rather, you need to use it with a realistic understanding of its strengths and weaknesses and figure out how to fill in some of the gaps that exist.
- Consider AI not as your only truth to replace clinical judgment, but rather, determine how clinicians can help to add the human perspective to best diagnose and treat a range of populations. “As the now old saying goes, AI won’t replace doctors, but doctors that use AI will replace those who don’t,” Kotecha stresses.
Find Ways to Expand the Data that Exists
Keep in mind that solving bias in health care won’t be a quick or an easy fix. Therefore, you need to be prepared to start out making small changes and to work with other key players over time in order to naturally begin to expand the data captured. This also requires advocating for more inclusive research efforts and supporting more equitable policies to ensure all populations access important healthcare services.
“The problems that currently exist in our health care system are complicated. There are ten little things that need to be addressed, so there’s not one fix to suddenly make the bias disappear. Rather, we need massive structural change that is carefully designed and thought through to make a real difference,” Kotecha says. This will require leaders who are equipped with the skills necessary to implement these technologies safely and effectively in health care organizations. They must also be committed to working toward a common goal that will ultimately help organizations implement AI to the full potential that exists.
Harvard T.H. Chan School of Public Health offers Responsible AI for Health Care: Concepts and Applications, Innovation with AI in Health Care, and Implementing Health Care AI into Clinical Practice, online programs that help medical and technology professionals improve health outcomes using AI.